Neural Machine Translation (NMT); it’s time we acknowledged it.
Globally Speaking predicted it as the big translation trend two years running, and Slator named May 2018 its busiest month yet, shortly after Amazon Translate joined the growing pile of NMT providers in April [1]. But what is it? What makes Neural Machine Translation so different from its predecessor, Statistical Machine Translation (SMT)? And how will it change how freelance translators and translation agencies work?
Life before NMT
As a language service provider, the dilemma of how to deal with machine translation creeping into the workplace is not new. The first real use of machine translation was way back in 1954, in the early days of the Cold War, when a computer was used to automatically translate sixty Russian sentences into English as part of the Georgetown-IBM experiment [2]. Several MT systems, such as rule-based and example-based machine translation, were used until Statistical Machine Translation (SMT) was developed in the 1990s. SMT analyses huge parallel corpora, both bilingual and monolingual, to create an appropriate translation of a phrase or term using word-based, phrase-based and syntax-based rules. This was the model that programs like Google Translate were using up until 2017, which offered and still offers an efficient and instant solution for “casual” translation needs. In cases like this, translation tools can be legitimately useful for providing a rough gist of the meaning of a foreign text instantly and for free, but they can have humorously incorrect results (You can see some of the best Google Translate fails here). When integrated into Computer-Assisted Translation (CAT) tools, SMT has also been one of the main machine translation models used by professional LSPs to automatically translate certain text types (at the request of the client, of course). Automated Translation (AT) and post-editing can increase productivity for particularly large, repetitive technical texts that require urgent translation, for example. The human post-editing of these texts has, therefore, been a common service offered by translation agencies and freelancers for years.
NMT—the new kid on the block
Over the past two years, NMT systems developed by tech giants like Facebook, Amazon, Microsoft and Google have been steadily replacing the old SMT systems. For example, in 2016, Google first announced GNMT, Google’s Neural Machine Translation system [3]. According to the technical report published alongside its launch [4], GNMT aimed to “improve the handling of rare words” by dividing words into a limited set of common sub-word units (“wordpieces”). The technical workings behind NMT are quite complicated to get your head around, but all you need to know is that it consists of neural networks that use ‘deep learning’ methods to mimic the human brain and produce more ‘fluent’ translations. SDL claims that its NMT system can learn the meaning of the text, enabling the machine to perform the translation task at a semantic level to produce naturally sounding target texts [6]. To explain how it works, NMT has often been likened to other uses of AI, such as self-driving cars in the automotive industry [7]. Here, a statistical approach would enable a car to drive well on a track, using the data from previous drivers who have driven down that road. However, a neural approach is less specific, as it enables a car to drive well on any road, using the data from different drivers or tracks. This type of artificial intelligence therefore learns by training data, rather than the software. This means that the data is the asset, rather than the complex code behind the program.
Will NMT be replacing humans any time soon?
While we’re not all rushing to replace human drivers with AI just yet, Slator predicts that NMT will drastically transform the translation industry, impacting over 600,000 linguists and 21,000 language service providers, as over 30-50% of all translations worldwide will be completed using NMT within one to three years [8]. However, there will, of course, always be instances where AI cannot translate with human-level accuracy and creativity, such as with word play, cultural references and literary text. Language lovers and writers will forever argue that a machine cannot reproduce style and subtlety in the same way as a human. Here are several more reasons why we will still need human input, despite NMT being more accurate and fluent than its predecessors:
1. NMT depends on the quality and availability of domain-specific data
The internet is a vast resource of continually updated bilingual material, meaning that more and more data is readily available to train NMT systems. While this content is often of a high quality, online content is not controlled. For example, in 2015, Google Translate, which learns by pulling in existing online translations, came under fire when the word “gay” was being translated into English as a variety of homophobic insults [9]. Serious errors like this are not intentional, but Google Translate is also notorious for its “Easter eggs”, where it spews out mysterious messages when gobbledygook is translated between specific language pairs. The most recent of these was a cryptic warning that read “Doomsday Clock is three minutes at twelve We are experiencing characters and a dramatic developments in the world, which indicate that we are increasingly approaching the end times and Jesus’ return” when users translated “dog” multiple times from Hawaiian into English. While these pranks or anomalies are entertaining, it just shows how sophisticated programs like GNMT can be manipulated and therefore lack the reliability of a human LSP. This is why companies like Facebook and Google then rely on their users (the humans!) to alert them about errors, provide translation feedback and improve their NMT in huge-scale projects.
Furthermore, empirical research led by Philipp Koehn and Rebecca Knowles, which was summarised in their paper “Six Challenges for Neural Machine Translation” [10], found that, because NMT is domain-specific, i.e. the data it learns from must correspond to the field being translated, it can “completely sacrifice adequacy for the sake of fluency”. A lack of resources can therefore result in worse quality. Text types and fields can also be hybrid, whereby genres, functions and style are fluid and overlap. Working with NMT therefore raises new challenges, as meaning and style can vary significantly between and even within texts, and the adequacy of the output varies from field to field.
2. There are special agreements between an LSP and its clients
The improved accuracy of NMT may be attractive to some clients looking for a cost-free translation solution, but clients must remember that whatever they enter into Google Translate, for example, then belongs to Google. This is an absolute no-go for sensitive content or proprietary texts, as the owner of the NMT service would then be free to use your precious written content as it pleases. Slator has suggested that one future solution for improving open-source NMT services would be to integrate blockchain, another rapidly developing technology, to pool translation memories [8]. Companies could then leverage their previously translated material when other companies pay for it, protecting their intellectual property rights. This may become an option in the future, but the trust, understanding and confidentiality built between an LSP and its clients certainly cannot be replicated elsewhere. Translation agencies and freelancers therefore need to emphasise and value these human relationships that make for successful projects.
3. NMT makes very specific errors
Of course, humans can make mistakes when translating or proofreading, such as typos and inconsistencies in terminology, which are easily spotted by using QA and terminology features in CAT tools. However, here at Web-Translations, we have been working with many of our linguists for years, meaning that we often use the same pairs of linguists who are used to proofreading each other’s work. Our linguists therefore know to look out for certain mistakes that another linguist might make. However, studies show that NMT can make significant errors that a human would never make, despite appearing more “fluent”. These include mistranslating rare words or proper nouns and ignoring the wider context, as NMT works better on shorter sentences (up to 60 words). A human post-editing a very fluent translation carried out by NMT may therefore accidentally overlook errors relating to accuracy.
Different errors also require varying levels of human intervention, so linguists would have to adapt to these new workloads. A study led by Adrian Probst [11] in 2017 compared the cognitive efforts of translators post-editing different error types in NMT- and SMT-translated texts by analysing the pause length prior to correcting errors. His research confirmed that there is a difference in pause length prior to post-editing certain error types yet there was no difference in pause time prior to editing errors between SMT and NMT. Despite NMT making fewer errors, linguists therefore need to be retrained in how to spot and efficiently post-edit the specific mistakes that NMT can make to ensure the same levels of quality.
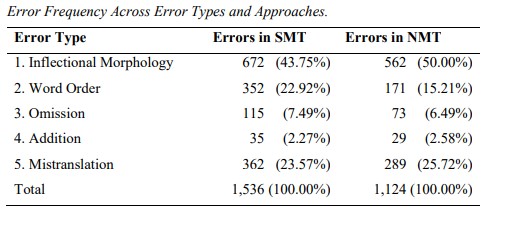
NMT makes fewer errors compared to SMT, but it’s still not perfect! (Table taken from “The Effect of Error Type on Pause Length in Post-Editing Machine Translation Output” by Adrian Probst, p.26)
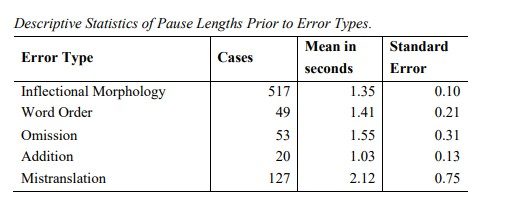
Linguists spend longer post-editing certain NMT error types (Table taken from “The Effect of Error Type on Pause Length in Post-Editing Machine Translation Output” by Adrian Probst, p.30)
4. Practicality and price
At the end of the day, these sophisticated new NMT systems can only be implemented by companies with a large budget to invest in the hardware required to train NMT and provide greater computational power to operate it. In terms of staff and training, smaller companies would also have to adapt to quicker turnarounds, lower pricing, high workloads and new QA measures. The reality of NMT creeping into most workplaces outside of huge companies like SDL is therefore quite unlikely for the time being.
The verdict
While the challenges facing NMT may reassure translators and translation agencies for now, we must be open to integrating this fast-developing technology into the translation workflow when the time comes. No matter how unwilling we are to admit that robots cannot replace human brains altogether, it is clear that they will become huge parts of our industry and new roles will be created for humans out of these technological developments. Vocational translation degrees and training programs should not only develop the translation skills of their students, but also train these future professionals to post-edit. As translators, we must defend our place yet overcome our fear of feeling obsolete by keeping up-to-date with tools, gaining new qualifications or accreditations and improving productivity. It is, therefore, in our interest to embrace this change and learn how to work alongside NMT and future automated translation solutions if we want to survive in the translation industry.
For more on how to sell yourself as a human translator, read our blog post:
References and links for further reading
[1] “Amazon and Lionbridge Share Stage To Market Neural Machine Translation” by Florian Faes: https://slator.com/technology/amazon-and-lionbridge-share-stage-to-market-neural-machine-translation/
[2] “A History of Machine Translation from the Cold War to Deep Learning” by Ilya Pestov: https://medium.freecodecamp.org/a-history-of-machine-translation-from-the-cold-war-to-deep-learning-f1d335ce8b5
[3] “A Neural Network for Machine Translation, at Production Scale” by Quoc V. Le & Mike Schuster, Research Scientists, Google Brain Team: https://ai.googleblog.com/2016/09/a-neural-network-for-machine.html
[4] “Google’s Neural Machine Translation System: Bridging the Gap between Human and Machine Translation”: https://arxiv.org/abs/1609.08144
[5] “State of the Art Neural Machine Translation” by SDL: https://www.sdl.com/ilp/language/neural-machine-translation.html
[6] “3 Reasons Why Neural Machine Translation is a Breakthrough” by Gino Niño: https://slator.com/technology/3-reasons-why-neural-machine-translation-is-a-breakthrough/
[7] “NMT: How SDL is Conquering the Most Challenging of all Artificial Intelligence Applications” by Mona Patel: https://blog.sdl.com/digital-experience/nmt-how-sdl-is-conquering-the-most-challenging-of-all-artificial-intelligence-applications/
[8] “Neural MT and Blockchain Are About to Radically Transform the Translation Market” by Ofer Shoshan: https://slator.com/sponsored-content/neural-mt-and-blockchain-are-about-to-radically-transform-the-translation-market/
[9] “Google Scrubs Google Translate of Offensive Gay Slurs” by Aaron Mamiit: https://www.techtimes.com/articles/29175/20150128/google-scrubs-google-translate-of-offensive-gay-slurs.htm
[10] “Six Challenges for Neural Machine Translation” by Philipp Koehn and Rebecca Knowles, p.28: http://www.aclweb.org/anthology/W17-3204
[11] “The Effect of Error Type on Pause Length in Post-Editing Machine Translation Output” by Adrian Probst (p.6): http://arno.uvt.nl/show.cgi?fid=144937
31 August 2018 12:54